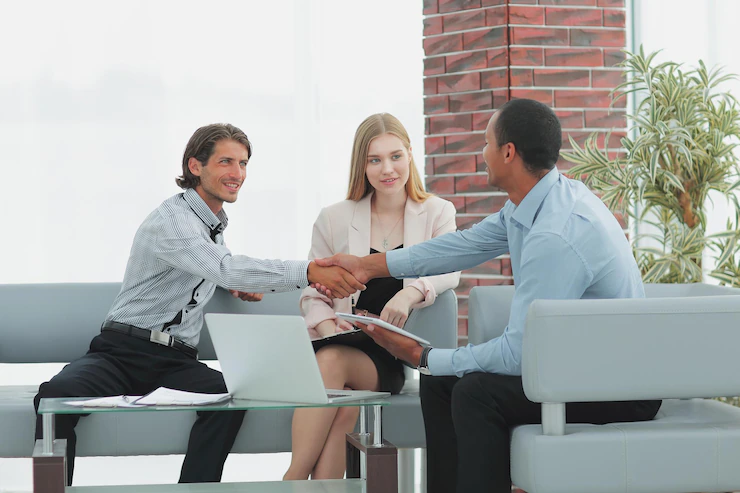
Product teams sometimes get wrapped up in day-to-day duties and neglect listening to what the consumer is saying. They are unable to collect customer experience, satisfaction, or loyalty data, to improve products and services, or lower churn rates. Moreover, some businesses don’t even get product feedback since they don’t have a feedback loop system in place. As a result, they continue to operate in the dark, failing to recognize customer needs. This is where sentiment analysis comes in to the picture with the help of ML models and deep neural networks.